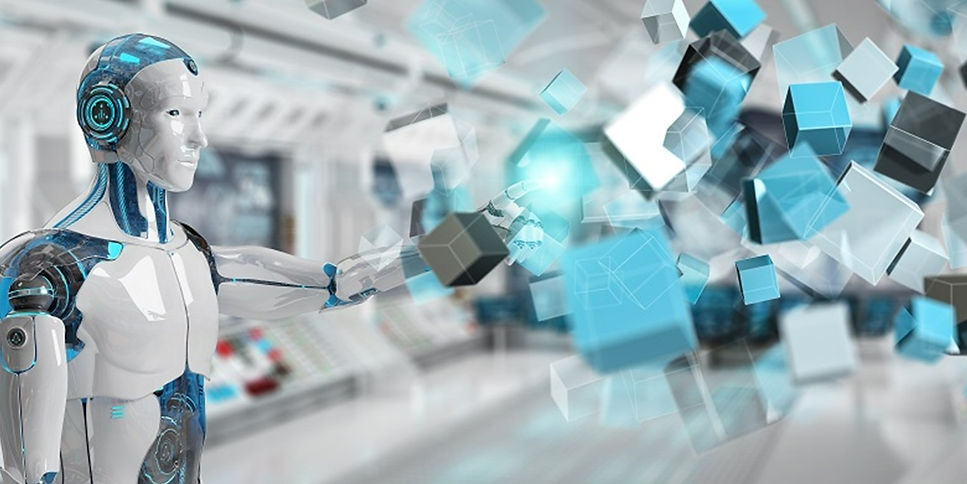
Getting the Best from Humans and Machines
This talk is about valuers and machines. It will range from the valuer’s unique obligation to safeguard the public trust, to the superficial gee-whiz view that seems to form around new technologies in the view of that same public.
​
I take the valuer’s viewpoint because I am one, and have been for 35 years. I am an engineer, and a sometimes philosopher. I practice real estate appraisal and business valuation, and specialize in the multidisciplinary space that involves both. I will try to put some difficult topics in context, and suggest ways to think about our role and the path forward that I hope will be useful for everybody in this room and the organizations you represent, but especially your members, who are having to hang onto a business that must confront a rapidly changing world. On the good news side, I believe moving through all this will be easier than we think. I am honored to have been asked by TEGOVA to address this topic.
​
I mentioned that we have an obligation to safeguard the public interest, and will keep coming back to this theme. This is the most inspiring thing to me about being in our professions. It is an honor, but also a heavy responsibility. We are nearly the only ones placed in this position. Everyone else in the investment, property ownership and every other community has a personal interest. Every transaction is investment value for the parties. Each has their special interests that they want to maximize. The banks want deal flow. The lawyer advocates for their client. Not wrong at all, it’s just the way it is.
​
There are only a few other professions charged with public protection, such as the judiciary, auditors, maybe some others. We are sometimes a problem for those with self-interests. We serve the public, not one side or the other. We are also indispensable. This is who we have chosen to be. All this only works if we are seen as credible. If our opinions are supported, transparent, and conform with all the requirements of good evidence for value. Our currency is trust. We must maintain that trust at all costs.
​
The need for trust has only been amplified over time. Financial engineering and various features of the law have acted to transfer risk from ‘too big to fail’ institutions to investors (including pension funds, municipalities, and many others consisting of or supported by the public), and ultimately through central banks and political institutions directly to the taxpayer. The incentive to manage risks must now be imposed by always-imperfect regulatory regimes. Internal incentives are vanishingly small. The public is the ultimate guarantor. We are here. There are not many lines of defense left.
​
Enter the machines
Technology produces lots of interesting stuff. All the time. I am an engineer, so I understand the fascination. Machines can provide great benefit to mankind, and can also do great damage. They have no moral sense. That must either be taught or imposed from outside. We must work with machines, now and especially into the future. But we must set the terms by which the machines must abide. Otherwise, they can take on the quality of Trojan Horses, corrupting our process to advance self-interested causes. We cannot let this happen.
​
The buzzword is “AI” for "Artificial Intelligence.” This encompasses a number of different technologies that have very different characteristics and with which we can interact in different ways. Some very helpful, some useful in some ways, and others either downright dangerous or worthless.
​
The simplest machines are algorithms; coded logic that accepts inputs and provides output(s). They have been in use for a long time. They are designed to perform specific tasks, and can be extremely useful tools for saving time, improving accuracy, and generally augmenting the skills of the user. Their functions can be known (if disclosed, of course), their theory understood. We have been using algorithmic models for years, and many more will be developed. No problem.
​
The next step is machine learning applied to algorithms whose functions are able to become more refined and sophisticated over time. The iPhone does this to learn how to recognize your face more and more efficiently. Learning can also be reprogrammed into algorithms by humans who simply learn how they work with their users and then modify their functions to make them better.
​
The appraisal industry has had automated valuation models (AVMs) for a long time. They are primarily algorithms, but I am sure they are evolving by adapting machine learning techniques.
​
Natural language processing has also been in use for some time. The idea is to help computers understand, process and generate speech as humans would. Even gauge emotion. Examples are Siri and Alexa, web search engines, spam filtering, spellchecking and text prediction.
​
Deep learning, neural networks, large language models and GPT all refine their ability to become proficient at some activity, but their performance is highly dependent on their training inputs. Enormous potential, but currently unable to do more than output statistically probable text and information. They routinely make mistakes now called “hallucinating.” (Nice try at spinning “wrong.”)
​
The worth of various AIs for valuation, particularly AVMs, is most strongly a function of their transparency. The more complex have their functions shrouded as ‘trade secrets’ by their creators. The machines cannot even tell you what they did if you ask them. This causes such AIs to be utterly worthless, no matter how accurate their predictions. If you can’t cross-examine a machine, it is no good to a valuer. At all.
​
A cyborg version
What about ‘valuer-assisted’ AVMs? Well, what about it? What might be the point? Oh yes. Pay the valuer less, because less work is involved, right? No, no, and no. What does the valuer have to do to check a machine’s output? An appraisal. The same work that would have to have been done absent the machine. So what has the machine contributed? Exactly nothing. An exception might be a transparent AVM whose data and processes could be reviewed. Fine. Then the valuer can perform a review, for perhaps a bit less. Unless the valuer changes the value. Then it’s an appraisal. No progress has been made.
​
“Valuer-assisted” AVM is sounding a lot more like asking the valuer to issue a fairness opinion; conclude that the AVM result is in a reasonable range. Such opinions are issued by large valuation firms and banks as part of M&A transactions. Mostly an insurance function, since the deal price is already done. The big difference is that such valuations cost hundreds of thousands, if not millions of euros. I’m pretty sure this isn’t the bank’s idea of “valuer-assisted” but from the valuer’s side it is exactly what they want. But the idea that it should be cheaper is abusive. Hard no.
​
It is interesting to note that a similar attempt is being made in Hollywood, where writers are on strike. The studios are attempting to use large language models instead of humans to write scripts. Of course, the scripts are not very good, so the plan is to hire the writers to do rewrites of the AI-generated ‘source material’. Rewriting can actually be more work than original writing, but it is paid at a lower rate. Same deal. Same problem. Same hard no.
​
The best of both
So what should determine the valuer’s relationship to the machines? In a word, judgment. The valuer possesses many years of experience, and has a brain that is optimized to make explanations from relatively small amounts of data. It is astonishingly good at this. Machines can explain nothing at all by themselves. They just generate statistically-probable outcomes. It seems an entirely worthy goal that we find ways to take advantage of the capabilities of both humans and machines.
​
We’ve commented on machine behavior so far. Human judgment is next. In my view, there are two types of judgment, the first of which is extraordinarily important and should be expanded. Let’s call that essential judgment. The second is a problem at best and extraordinarily harmful at worst. Let’s call that made-up judgment.
​
Essential judgment is just that; a human quality that is really the core of the valuation process. It is the interface between the appraisal process and the real world, in which the valuer gathers and interprets facts & circumstances of the property/ business/ markets/ parties/ history and future expectations in terms that analytical models and processes can use. It is where experience shines and wisdom shows itself. It often leads the valuer to discover conditions that can hugely affect the outcome but would otherwise remain hidden (until reality intervenes and the expected consequences are realized). The appraisal must include explanations of such discoveries and their effect on value. Good explanations are transparent, and can be cross-examined. They do not require mountains of data. Such are the capabilities of the human mind “which by dint of language, in the words of Wilhelm von Humboldt, can make ‘infinite use of finite means,’ creating ideas and theories with universal reach.”
​
Made-up judgment is also just that; an invention by the valuer. This is not a bug so much as a feature of valuation processes that are by their nature opaque and lacking in relevance. Such processes have ended up in professional bodies of knowledge because they do have very germane and important applications. They serve to discover investment behavior and create observations that can be used to good effect. But they are hard to learn and apply. Some that come to mind may be found mostly in the sales comparison/market approach, and include public guideline company and direct market data methods, anything involving restricted stock (for marketability discounts), transactions involving fractional interests in real estate, and property sales themselves. (Property sales are less prone to made-up judgment for conforming properties in very active markets.)
​
Creating transparency with these types of data can certainly be produced, but it is a lot of work. To the extent that the work is not presented clearly, characteristics of the property, purpose of the transactions, motivations of buyer and seller, and a great many other elements are just assumed or ignored. On the one hand, valuation is about using the data we have. On the other hand, data that is chronically opaque sets up a credibility problem for the valuation and its conclusion. These processes are accepted by non-valuers for the simple reason that more-or-less everyone owns a house or wants to. Comparisons with other house sales makes intuitive sense. But valuers really do know that the ‘devil is in the details,’ and the devil wins more often than we would like to believe.
​
Made-up judgment also has a much darker side, one that facilitates bad behavior. Bad actors, hustlers and outright fraudsters can be found in all professional fields, of course. But biasing an opinion of value is one of the greatest sins that can be committed by a valuer whose obligation—and the public’s legitimate expectation—is that the valuer should be objective and neutral. I noted earlier that nearly everyone but the valuer has a personal bias that they want to be realized, whether it’s simply maximizing their benefit in a transaction, stealing something from their investment partners in a court action, or cleverly defrauding the government of taxes owed. Again, the human desire is simply a fact, but valuers are faced with blocking such goals and offering only neutral, objective opinions of value. It can take a lot to resist, and some simply don’t. They go along. In fact, their reputation for concluding favorable values leads others (thinking lawyers here) to hire them for that specific reason. Valuation is usually a complex process, and a good con artist can deliver persuasive stories. Some get paid very high rates for just that. They are usually known to the valuation community. Their work continues.
​
Opportunities for bad judgment are readily available in valuation. They are an unintended but predictable consequence of imprecise methods and obscured information in the data. But now that we are considering useful connections between valuers and machines, I propose that we use machines, algorithms in particular, to eliminate opportunities for made-up judgment to the greatest extent that we can. If taken on as a professional imperative, this effort could go a long way to supporting our credibility and service to the public that is our charge.
​
Modeling the models
I am not entirely sure how to apply an algorithmic solution to understanding and selecting, say, business transaction data from a large compiled dataset such as DealStats. A lot of information we would like is just not included in the data. Real estate transactions have a similar problem, but real estate appraisers are used to interviewing parties to the transaction and gaining a good understanding. This cannot always be done, however, and the basic concern remains.
​
I have created an algorithmic application that addresses nearly every potential issue raised above, though, and hope that it can serve as a model of algorithmic solutions for fostering the essential judgment we need from valuers as well as blocking opportunities for bad judgment that are otherwise set up obscured data and vulnerable models. Building the algorithm forced me to work through these specific issues in detail. In the end, it is really not complicated at all.
​
My practice specialty, and area of algorithm application, is valuing fractional interests in real estate (partnerships, LLCs, tenancy-in-common and related ownership circumstances). It is a multidisciplinary niche that is uncommon in some valuation markets. But it has suffered greatly from blind spots that are created when knowledge generated by, say, the real property appraisal discipline must be understood by the business valuer. Or the reverse. Multidisciplinary valuation is quite a long topic on its own, but suffice it to say that guidance on both process and the correct application of judgment has been sorely needed for a very long time.
​
The core of the application is the income approach, because it is inherently quantitative, it is mostly unchanged in appearance and function when used in different disciplines, and because its operations are easily made transparent. It turns out that the income approach allows for a much more complete, logical and reliable valuation process than any of the alternatives. The facts and circumstances of the case are interpreted as influencing the input variables (risk elements, growth rates, holding period) for conventional discounted cash flow and other models. It is most convenient that these elements relating to the fractional interest position itself are also represented in the underlying real estate asset itself, whether income-producing or not. The algorithm simply executes the models using established and agreed techniques that I have demonstrated in full detail. Both the process and the databases it includes are objective and tightly connected with the inputs. There is no interpretive “wiggle-room” that can be exploited by bad actors to bias the results.
​
Essential judgment is guided directly by the application. A lot of guidance is necessary because of the many blind spots generated by joining two disciplines. The application user is guided step-by-step through a long series of questions, broken down into key divisions (the real estate, the entity and its financials, risk attributed to management by others and the ability of the interest-holder to influence outcomes, legal exit options, and risk attributed to an inability to exit the position—with its share of the underlying equity—for a time).
​
The valuer’s judgment results in concluded growth rates, modifications to risk rates and financial pro formas that would be expected based on market observations. This reaches deep into the experience and training of the user, whether real property appraiser or business valuer, and provides detailed guidance in the form of background, theory and references for those elements with which the user might not be familiar (the multidisciplinary blind spots). This list is only complete because I have been practicing in this exact mixed field for almost thirty years, and am fully qualified and experienced in both disciplines. The guidance and algorithm are readily usable by valuers in either discipline.
​
The result of the valuer’s input and the algorithm’s computations is output as a list of the questions, the valuer’s answers (which amount to explanations of specific facts/circumstances considered and variables selected), along with internal formulas and computations. Everything is transparent. This summary is then crafted into a report by the valuer. Maximum use is made of the valuer’s training, experience and ability to explain his or her conclusions, step-by-step. Essential judgment is highly prized. There are no opportunities for made-up judgment to change the result that wouldn’t be put directly on display, keeping opportunities for misuse low or closed off entirely.
Conclusions and the way forward
Valuers are faced with an existential challenge, particularly with respect to collateral lending. Nothing new there, but the rise of the machines is making the challenge much more immediate. We must respond by understanding our value and then telling everybody. We need support of a public that is too often impressed with technology that carries a ‘gee whiz’ factor. We are charged with protecting the public trust.
​
The incentives to game the system are enormous and unrelenting. The need for objective opinions of value is great, and the benefits to the public are simple. Systematic failures are often forgiven if the perpetrators are willing to say “whoops.” The public (taxpayers) end up with the bill.
​
Machine-generated values are not necessarily a panacea, and may contribute nothing at all. The danger for our professions is that the public believes machines to be intelligent and that they have the same capacities as humans. They do not. Machines need to work with humans, not replace humans, particularly in valuation.
​
Getting the best of from both valuer and machine is not only possible, it exists now, as PrimusPVX. The application fosters essential judgment and blocks made-up judgment, is transparent and can be adopted to perform complex, multidisciplinary valuations quickly and easily.
​
​
Dennis Webb
​
Copyright © Dennis A. Webb, 2023, All rights reserved.